Flying sensors have revolutionized crop yield forecasting, offering you seven powerful techniques to maximize your farm's productivity. You'll benefit from multispectral imaging analysis to detect plant stress, thermal mapping for pinpointing problem areas, and LiDAR for precise 3D crop modeling. RGB imagery combined with AI processing provides quick, accurate assessments, while hyperspectral data reveals hidden crop issues. Time-series vegetation index monitoring tracks growth patterns over the season, and machine learning integration enhances prediction accuracy by combining multiple data sources. These cutting-edge methods empower you with unprecedented insights into your fields' health and potential yield. Exploring these techniques further will reveal even more ways to optimize your agricultural operations.
Multispectral Imaging Analysis
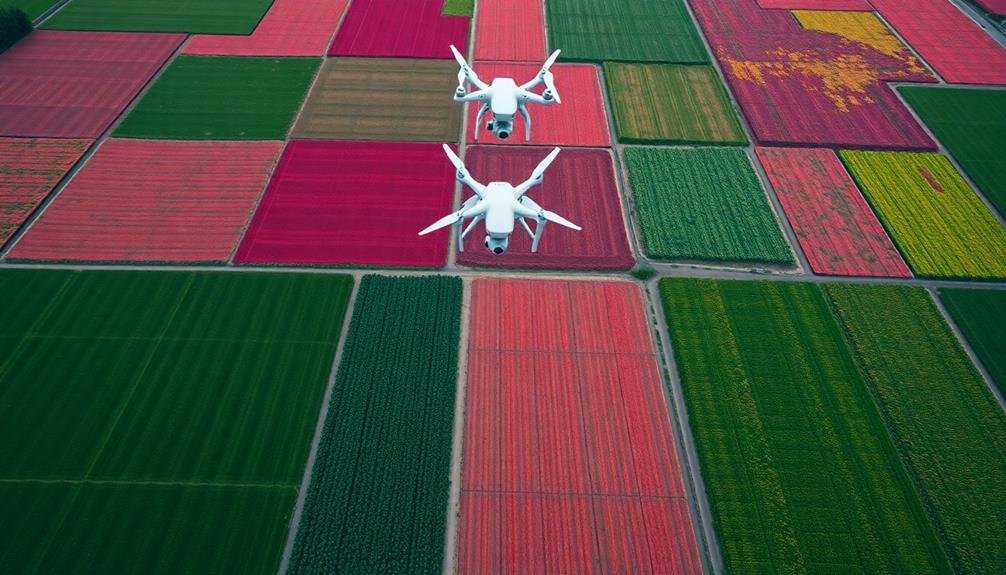
Through multispectral imaging analysis, you can gain valuable insights into crop health and potential yield. This technique uses specialized cameras mounted on drones or satellites to capture images across multiple light wavelengths. You'll be able to detect subtle variations in plant reflectance that aren't visible to the naked eye.
By analyzing these images, you'll identify key indicators of crop stress, nutrient deficiencies, and disease. The near-infrared (NIR) band is particularly useful for evaluating plant vigor and biomass. You'll also use the red-edge band to detect early signs of chlorophyll content changes, which can indicate plant health issues before they're visible.
You'll process the collected data using specialized software to create vegetation indices like NDVI (Normalized Difference Vegetation Index). These indices help you quantify crop health and predict potential yields more accurately. By combining multispectral data with other factors like weather patterns and soil conditions, you'll develop thorough yield forecasts.
This technique allows you to make timely management decisions, such as targeted fertilizer application or pest control measures. You'll optimize resource use, reduce crop losses, and ultimately improve your overall yield predictions.
Thermal Mapping for Stress Detection
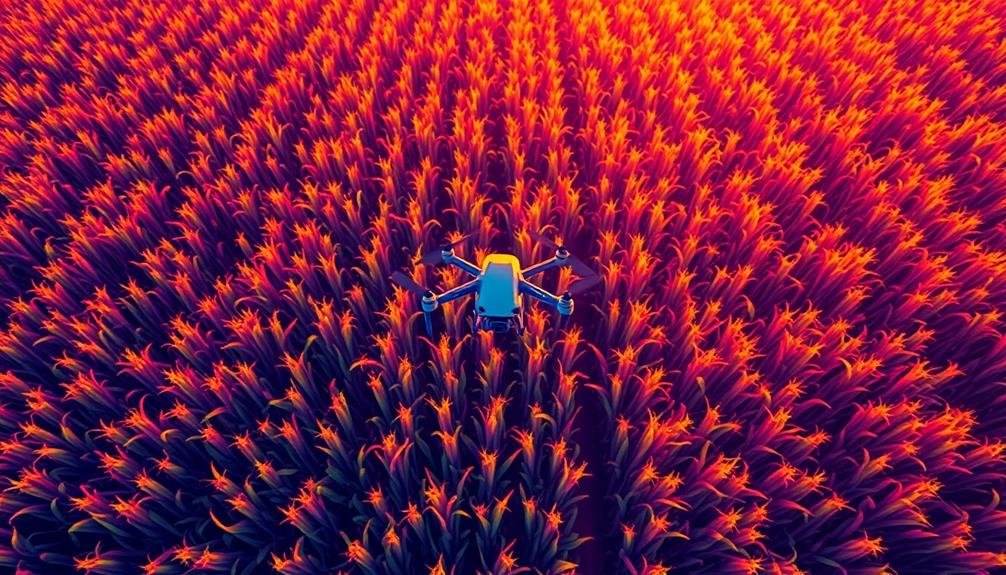
While multispectral imaging provides valuable insights, thermal mapping offers a unique perspective on crop stress detection. You'll find that thermal sensors on flying platforms can capture temperature variations across your fields, revealing areas of plant stress before they're visible to the naked eye.
Thermal mapping works by detecting infrared radiation emitted by plants. When crops are under stress, their temperature often increases due to reduced transpiration. You can use this data to identify:
Stress Factor | Temperature Change | Potential Cause |
---|---|---|
Water Stress | Increase | Drought, Poor Irrigation |
Disease | Increase | Pathogens, Pests |
Nutrient Deficiency | Varied | Soil Imbalances |
By incorporating thermal mapping into your crop monitoring strategy, you'll be able to detect stress early and take targeted action. This proactive approach can lead to improved crop management, reduced input costs, and ultimately, higher yields.
You'll want to combine thermal data with other sensor information and ground-truth observations for the most accurate stress detection. Remember, environmental factors like time of day and weather conditions can affect thermal readings, so it's essential to account for these variables when interpreting your data.
LiDAR-Based Canopy Height Measurement
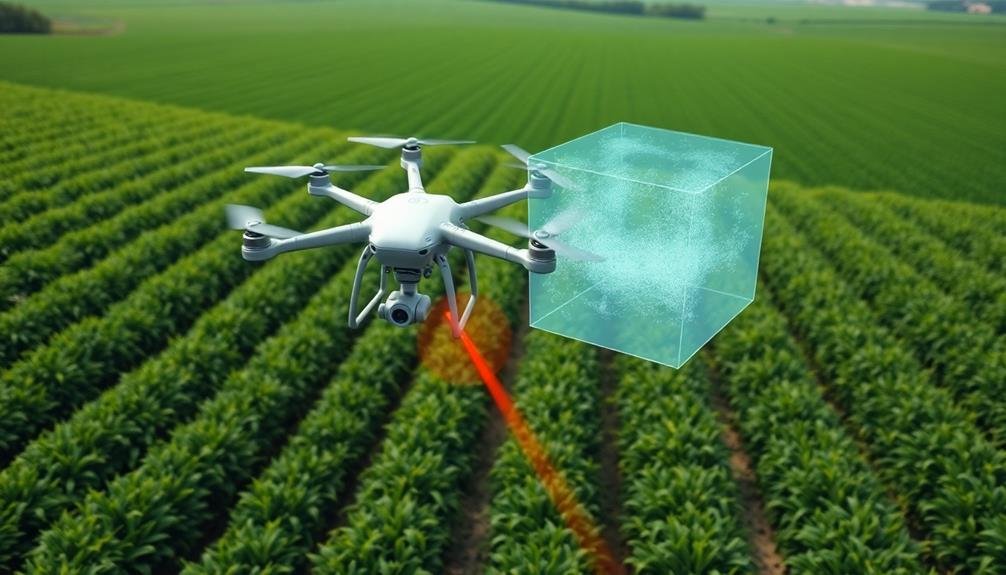
Moving beyond temperature-based stress detection, let's explore another advanced technique in crop yield forecasting. LiDAR-based canopy height measurement is a powerful tool that uses laser technology to create precise 3D models of crop structures. You'll find this method particularly useful for evaluating plant growth and predicting potential yields.
LiDAR sensors mounted on drones or aircraft emit laser pulses that bounce off the crop canopy, measuring the time it takes for the light to return. This data creates a detailed point cloud, representing the crop's height and density. You can use this information to estimate biomass, leaf area index, and overall crop health.
By comparing LiDAR data over time, you'll track growth rates and identify areas of stunted development. This allows for targeted interventions, such as adjusting irrigation or applying fertilizers. The high resolution of LiDAR data also helps you detect variations within fields, enabling precision agriculture practices.
You'll find LiDAR especially valuable for tall crops like corn or orchards, where traditional methods struggle. It's not affected by shadows or varying light conditions, ensuring consistent measurements throughout the growing season.
RGB Imagery and AI Processing
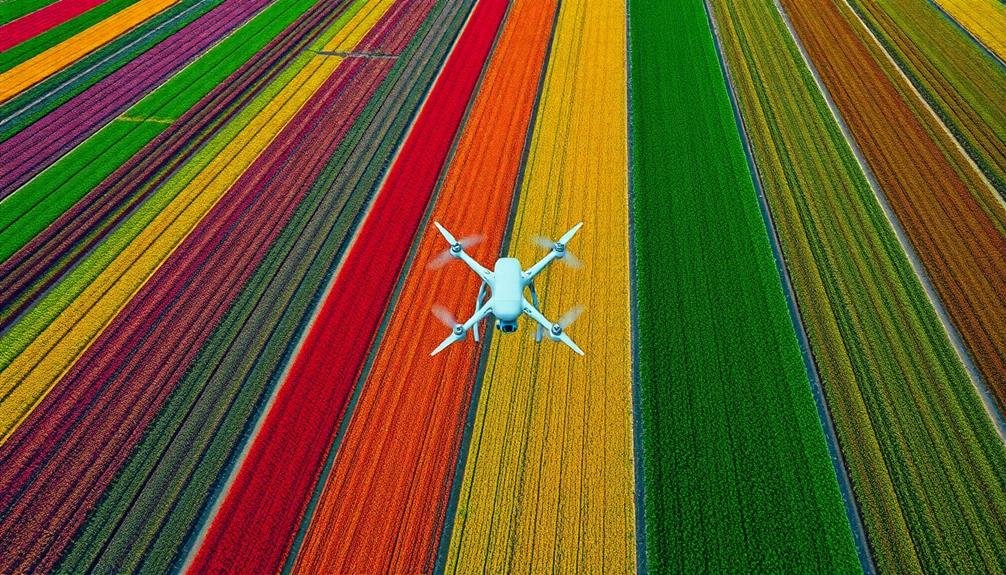
RGB imagery combined with artificial intelligence (AI) processing represents a game-changing approach in crop yield forecasting. You'll find that this technique leverages high-resolution images captured by drones or satellites, which are then analyzed using sophisticated AI algorithms. These algorithms can detect subtle variations in crop color, density, and growth patterns that might be imperceptible to the human eye.
By utilizing machine learning models, you're able to process vast amounts of data quickly and accurately. These models can identify key indicators of crop health, such as chlorophyll content, leaf area index, and early signs of disease or pest infestation.
You'll appreciate how AI can also factor in historical yield data, weather patterns, and soil conditions to provide more precise yield predictions.
As you implement this technology, you'll notice significant improvements in your forecasting accuracy. You can use RGB imagery and AI processing to make timely decisions about irrigation, fertilization, and pest management.
This approach allows you to optimize resource allocation and potentially increase your overall crop yield. It's a powerful tool that's revolutionizing the way you approach agricultural planning and management.
Hyperspectral Data Collection and Interpretation
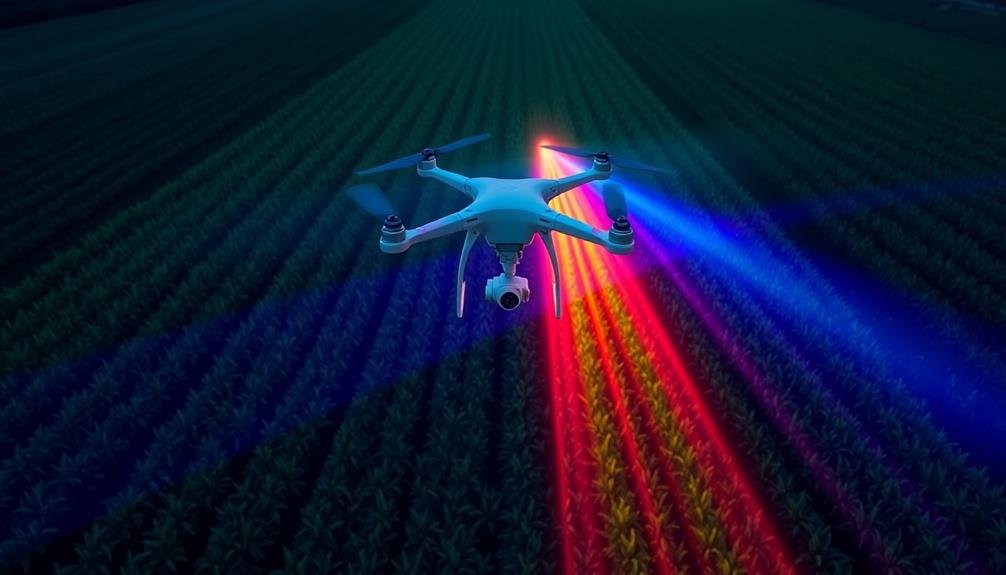
Delving deeper into advanced crop monitoring techniques, hyperspectral data collection and interpretation offer unprecedented insights into plant health and yield potential.
You'll find that hyperspectral sensors capture data across hundreds of narrow spectral bands, providing a detailed spectral signature for each plant.
To leverage this technology, you'll need to equip your drones with hyperspectral cameras. These sensors collect data on light reflectance across the electromagnetic spectrum, including visible and near-infrared wavelengths.
You'll be able to detect subtle changes in plant physiology, nutrient content, and stress levels that aren't visible to the naked eye or standard RGB cameras.
Interpreting hyperspectral data requires specialized software and expertise.
You'll use algorithms to analyze spectral signatures and identify key indicators of crop health. This data can help you pinpoint areas of nutrient deficiency, pest infestations, or water stress before they become visible symptoms.
Time-Series Vegetation Index Monitoring
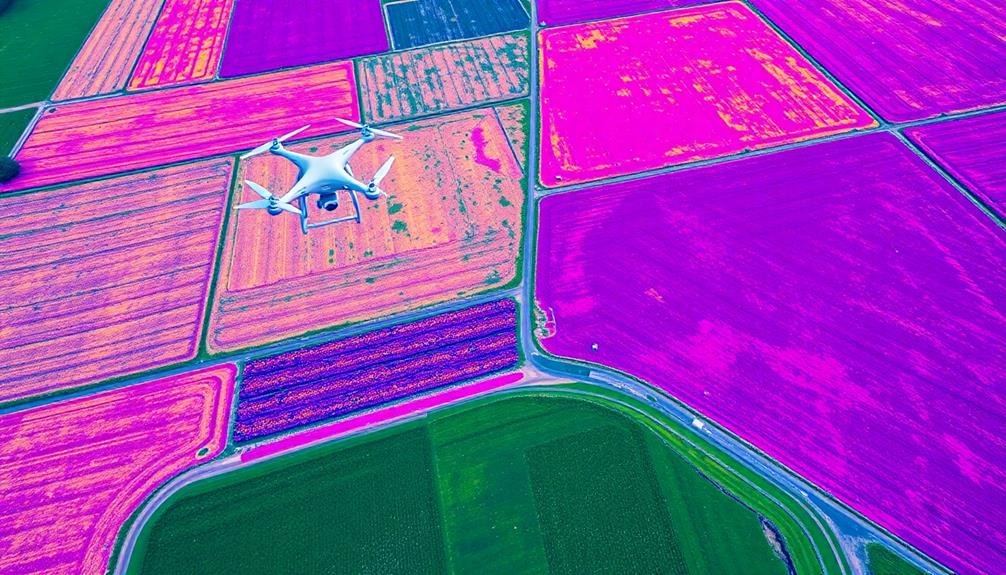
Consistency is key when it comes to time-series vegetation index monitoring. You'll need to regularly collect data throughout the growing season to track changes in crop health and development. This method involves using vegetation indices like NDVI (Normalized Difference Vegetation Index) or EVI (Enhanced Vegetation Index) to assess plant vigor and biomass over time.
To implement this technique effectively, you should:
- Establish a fixed flight schedule, ideally weekly or bi-weekly.
- Maintain consistent flight altitudes and image resolutions.
- Use the same sensors and calibration methods for each flight.
- Process images using standardized algorithms and software.
By analyzing the time-series data, you can detect trends, anomalies, and patterns in crop growth. This information helps you predict yield potential, identify stress factors, and make informed management decisions. You'll be able to spot areas of concern early, allowing for timely interventions.
Remember to take into account environmental factors like weather conditions and soil moisture when interpreting the data.
Integrating this information with ground-truth data and historical yield records will further enhance your forecasting accuracy.
Machine Learning Crop Model Integration
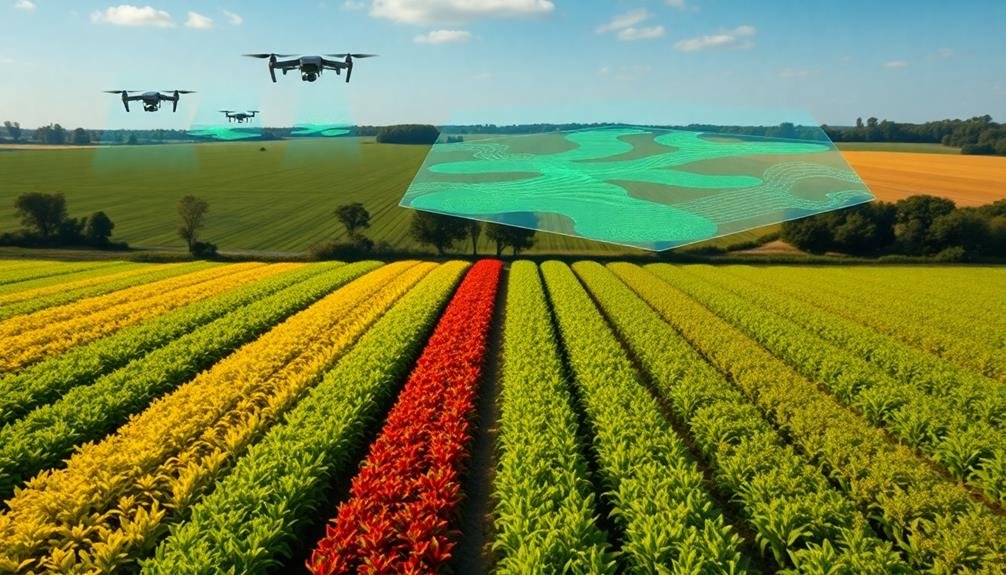
Machine learning algorithms are revolutionizing crop yield forecasting by integrating traditional crop models with advanced data analysis techniques. You'll find that this integration allows for more accurate predictions by combining the strengths of both approaches. Traditional crop models rely on physiological and environmental parameters, while machine learning excels at identifying complex patterns in large datasets.
When you're using this integrated approach, you'll input data from various sources, including satellite imagery, weather stations, and soil sensors. The machine learning algorithms will then process this information alongside the crop model's predictions, refining the forecast based on real-time data. You can expect improved accuracy in predicting yields, as well as better insights into the factors affecting crop performance.
You'll benefit from the ability to handle non-linear relationships and interactions between variables, which traditional models often struggle with. This integration also allows for continuous learning and adaptation as new data becomes available.
Frequently Asked Questions
How Do Weather Conditions Affect the Accuracy of Flying Sensor Data Collection?
Weather conditions greatly impact your flying sensor data accuracy. Wind can disrupt flight paths, rain interferes with sensors, and extreme temperatures affect battery life. You'll need to plan flights carefully and consider atmospheric conditions for reliable results.
What Are the Regulatory Requirements for Using Drones in Agricultural Monitoring?
You'll need to follow FAA regulations for commercial drone use, including registering your drone, obtaining a remote pilot certificate, and adhering to flight restrictions. You're also required to maintain visual line-of-sight and respect privacy laws.
How Cost-Effective Are Flying Sensors Compared to Traditional Crop Yield Forecasting Methods?
You'll find flying sensors are often more cost-effective than traditional methods. They cover large areas quickly, provide real-time data, and reduce labor costs. However, initial investment in equipment and training can be significant. Consider your specific needs carefully.
Can Flying Sensors Detect Crop Diseases Before Visible Symptoms Appear?
Yes, flying sensors can detect crop diseases before visible symptoms appear. They'll capture subtle changes in plant health using multispectral imaging and thermal sensors. You'll get early warnings, allowing you to intervene quickly and prevent widespread damage.
What Is the Typical Turnaround Time for Processing and Analyzing Flying Sensor Data?
You'll typically see flying sensor data processed and analyzed within 24-48 hours. It depends on the data volume, processing power, and analysis complexity. Some automated systems can provide near real-time results for quick decision-making.
In Summary
You've explored cutting-edge techniques for crop yield forecasting using flying sensors. From multispectral imaging to machine learning integration, these methods offer powerful insights into your fields' health and potential yield. By adopting these technologies, you'll make more informed decisions, optimize resource use, and potentially increase your crop yields. As precision agriculture evolves, staying current with these innovative approaches will give you a competitive edge in modern farming.
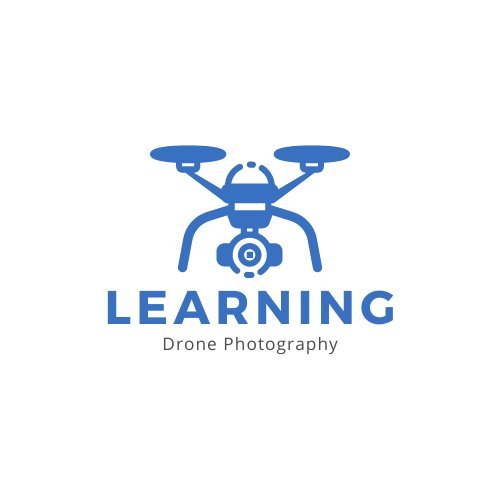
As educators and advocates for responsible drone use, we’re committed to sharing our knowledge and expertise with aspiring aerial photographers.
Leave a Reply